When it comes to AI, it’s not easy to keep track of things – especially when terms that keep popping up in this context are unclear. We want to help you understand the basic terms and explain what they mean.
What are the different AI approaches?
Reactive AI
Reactive AI is the “weakest” form of AI and we come across it, for example, when a simple robot puts parcels away in the warehouse. Its aim is to complete tasks based on information – without understanding the context, incorporating previous experience or gathering experience, as there is no memory. The systems are therefore not capable of learning.
Generative AI
Whether consciously or not, most of us have probably already touched on the topic of Generative AI. After all, the most prominent tool that uses Generative AI is ChatGPT. But it is by no means the only one: whether Google Gemini, Anyword, Runway or Suno – the tools all have one thing in common: as the name suggests, they are all about generating things: texts, images, audio files, videos or similar. For example, texts on websites, answers to customer inquiries or program codes can be created. The basis for this is content that is already available – well trained by the AI.
Predictive AI
Again, the name already helps us: predictive AI is about making predictions about the future – for example, focusing on business figures, the conditions of machines, health risks or logistics chains. Sales managers can find out which customers might cancel their contracts, engineers which components might cause problems, logistics managers how long various delivery routes will take and doctors which patients are at risk of developing a certain illness. This information is intended to help them make decisions.
The idea of predictive AI is not new. What is new, however, is the available computing capacity, which makes predictive AI accessible and increases its added value. Both historical data and real-time data can be used in the calculation.
Prescriptive AI
Prescriptive AI goes one step further. Here, users receive a suggestion for the best course of action. This would also tell sales managers how they can retain customers, engineers how a machine can best be repaired, logistics managers which route is the best and safest, and doctors which treatment method promises recovery.
While predictive AI “only” determines probabilities, prescriptive AI also makes recommendations and takes a company’s requirements into account.
Self-learning AI
Self-learning AI also reveals in its name what it can do – and that is quite a lot: here, the AI system should learn over time, acquire knowledge and adapt – without explicit programming being necessary.
This should happen through “try and error” or “learning by doing”. The AI should observe the effects of certain actions and fill gaps by recognizing patterns and drawing conclusions from them. The AI does not learn English at school for 10 years, but is thrown into the deep end and made to learn by moving to the USA.
What AI language models do exist?
Large Language Models
Large Language Models (LLM) are powerful models that process and connect countless data, understand and reproduce our language. They can handle complex instructions and generate content by taking into account the probability with which individual phrases/sentences follow one another. This enables them to perform tasks for which they have not been explicitly trained. ChatGPT is again an example of such an LLM model.
Two things are important with LLMs: firstly, the truth of the results must always be checked. With large language models, we can be confronted with hallucinations, i.e. untrue statements. Secondly, when deciding on a model, it should always be checked whether an LLM model – and therefore large computing capacities – are actually necessary.
And this brings us to the next type of language model.
Small Language Models / Short Language Models
As the last sentence and the name suggest: Small or Short Language Models (SLM) are smaller models. They involve much less data and therefore require significantly less computing power, are cheaper, can be trained more easily and can be provided more quickly. They can map very specific use cases, be fine-tuned for them and deliver better results. To stick with the chatbot example: An AI assistant on the website of a research laboratory does not need to be able to write poetry about nature. However, it must be able to make precise statements about the research results on a topic.
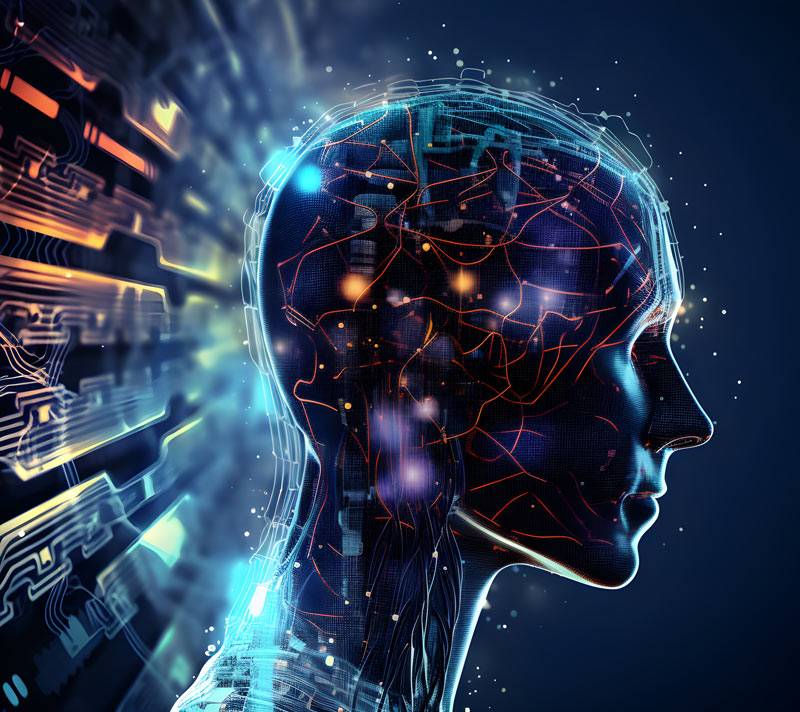
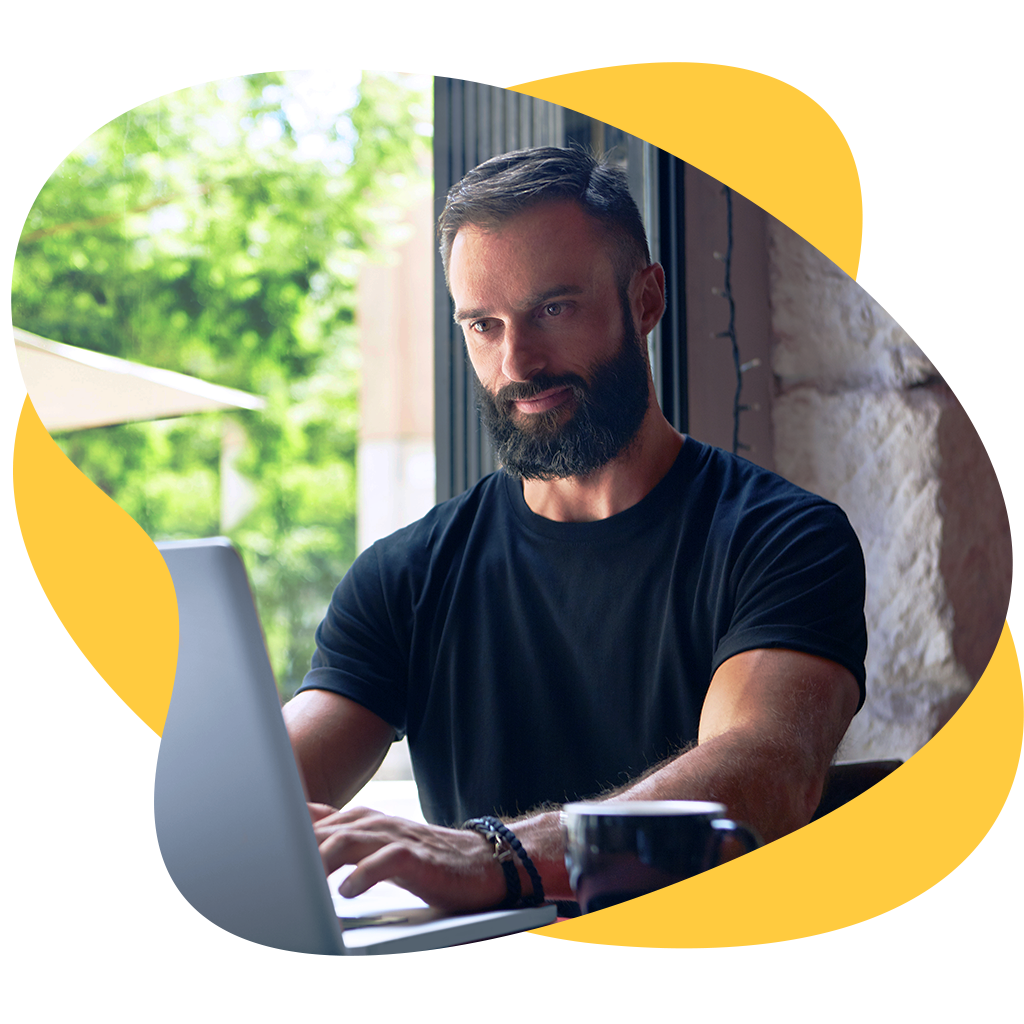
Get in touch with us
We can not only help you understand the various AI terms, but also have the knowledge of how Artificial Intelligence can be applied and create added value.